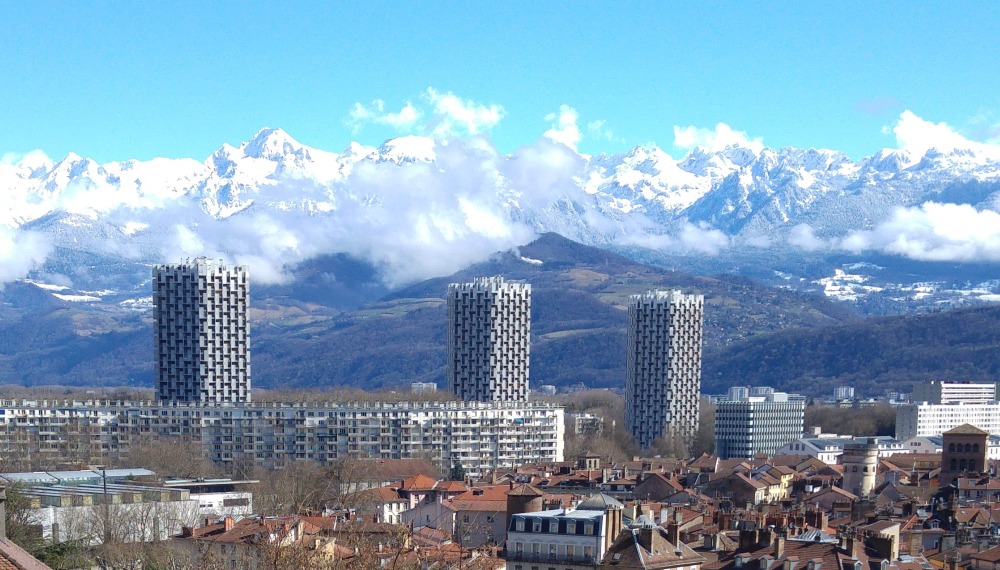
Dennis Bray, University of Cambridge Protein Networks versus Microchips
Cells are built up of molecular circuits that perform logical operations, analogous in many ways to electronic devices but with unique properties. Proteins and other molecules act like miniature transistors to guide the biochemical processes of a cell. Linked into huge networks they form the basis of all of the distinctive properties of living systems. However, as illustrated by our work on bacterial chemotaxis, the simple form of behaviour in which bacteria smell and swim towards distant sources of food, living circuitry differs in fundamental respects to silicon devices. It has unique features such as a highly malleable internal architecture and an existence of a multitude of molecular states that we cannot yet reproduce. The chemical states of a cell encode its past experiences and allow it to anticipate future conditions. Predictions underlie the goal-oriented movements of all animals, even single cells, and form the basis of brain function in humans.
Guillaume Beslon, INSA-Lyon Analyzing the past to understand the present: How evolution shapes biological systems?
All biological systems have an evolutionary origin: they acquired their extant structure and properties by the repeated action of variation and selection. But the evolutionary process, qualified of “tinkering” by François Jacob in 1977, may favor some specific structures and disfavor some. Understanding where evolution is the most likely to go could thus be very helpful to decipher the structure of extant biological systems. Yet, deciphering the structure of extant systems is necessary to understand where evolution has gone! To break this vicious circle, we propose to use in silico experimental evolution to evolve digital organisms. Being simulated, these organisms are perfectly known as well as their evolutionary history. We can thus use them to understand what are the evolutionary rules that shaped their structure and complexity. In this talk, I will illustrate the power of in silico experimental evolution using the aevol software (www.aevol.fr) and focus on the evolution of genomes and genetic networks. By evolving organisms in environments of various complexities I will show that, at least in in silico models, the complexity of an organism (size of the genome, number of genes, size of the regulation networks) may be more linked to its evolutionary dynamics than to its need to adapt to a complex environment. In other words, the structural complexity of a biological system may be independent of its functional complexity.
Albert Goldbeter, Université Libre de Bruxelles *Modeling the cell cycle by differential equations and cellular automata
To gain insight into the dynamics of this major cellular process we developed a detailed computational model for the network of cyclin-dependent kinases (Cdks) driving progression along the successive phases M (mitosis), G1, S (DNA replication) and G2 of the mammalian cell cycle. The model is described by a system of 39 nonlinear, coupled differential equations. A reduced, skeleton model containing only 5 ordinary differential equations yields similar results. The analysis of the models shows how the balance between cell cycle arrest and cell proliferation is controlled by growth factors (GFs) or by the levels of activators (oncogenes) and inhibitors (tumor suppressors) of cell cycle progression. Supra-threshold changes in the level of any of these factors can trigger a switch in the dynamical behavior of the Cdk network corresponding to a bifurcation between a stable steady state, associated with cell cycle arrest, and sustained Cdk oscillations corresponding to cell proliferation. To further study the dynamics of the cell cycle we developed an automaton model in which each cellular automaton switches stochastically between the sequential phases of the cell cycle, which have durations distributed around mean values. This approach is well suited to studying how the fractions of cells in a given cell cycle phase evolve in a cell population. The automaton model can be used to probe the existence of optimal schedules of circadian administration of anticancer drugs that target cells in a particular phase of the cell cycle.
Linda Petzold, University of California, Santa Barbara Functional Network Inference of the Suprachiasmatic Nucleus
In the mammalian suprachiasmatic nucleus (SCN), noisy cellular oscillators communicate within a neuronal network to generate precise system-wide circadian rhythms. Although the intracellular genetic oscillator and intercellular biochemical coupling mechanisms have been examined previously, the network topology driving synchronization of the SCN has not been elucidated. This network has been particularly challenging to probe, due to its oscillatory components and slow coupling timescale. In this work, we investigated the SCN network at a single-cell resolution through a chemically induced desynchronization. We then inferred functional connections in the SCN by applying the maximal information co-efficient statistic to bioluminescence reporter data from individual neurons while they resynchronized their circadian cycling. Our results demonstrate that the functional network of circadian cells associated with resynchronization has small-world characteristics, with a node degree distribution that is exponential. We show that hubs of this small-world network are preferentially located in the central SCN, with sparsely connected shells surrounding these cores. Finally, we report on our progress in developing a novel method to infer the directionality of the network.